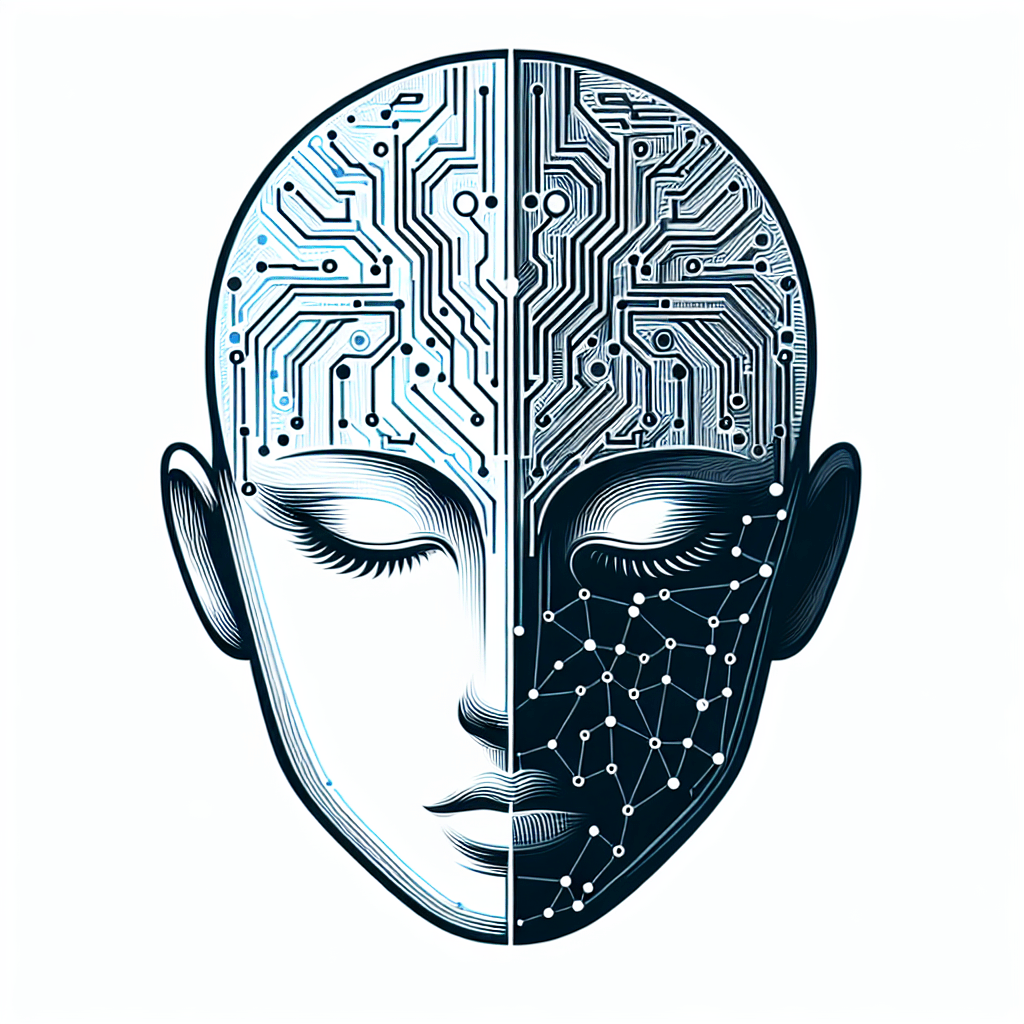
In the future, it’s likely that only a few companies will have the necessary resources to build and maintain large language models (LLMs) like GPT-3. However, according to Sam Altman, the next decade may witness the emergence of many companies building a “second layer” on top of these models, with valuations surpassing a billion dollars.
The concept of a “second layer” refers to companies built on top of base LLMs but with specific training, enabling the generation of business intelligence for specific industries and domains of knowledge. This approach could lead to the development of industry-specific solutions based on data related to legal aspects, procedures, specifications, etc.
To generate value, it’s necessary to move from theoretical models to production-level accuracy. This shift requires investment in data labeling and development. Custom-trained models consistently outperform their generic counterparts, such as ChatGPT, as demonstrated by studies.
The Importance of Data
OpenAI emphasizes the need for at least 100 labeled examples per class for effective fine-tuning training. This highlights that data development is not a mundane task; it initiates a powerful cycle.
The more fine-tuning occurs, the more adaptable and powerful the model becomes, shifting the focus from the base model to continuous refinement.
Therefore, this “second layer,” characterized by data development and fine-tuning, is where the true value of AI materializes: owning and mastering data is key to boosting AI capabilities.
GPT-You Personal Assistants
Additionally, there is considerable hidden value in the personal customization of GPT models to serve as personal assistants. These models present strong entry barriers, depending on the user’s relationship with the machine in environments with millions of captive users, as seen in the cases of Apple or Google.