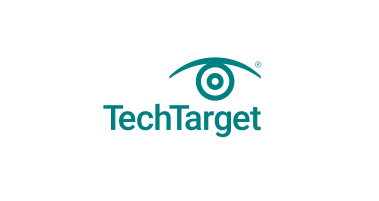
Understanding Data Poisoning
In the world of Artificial Intelligence (AI) and Machine Learning (ML), a predominant method of achieving system compromise is through data poisoning. This attack involves manipulation of ML model’s training data to corrupt its behavior and generate skewed or harmful outputs. The broader application of AI tools, proliferated by the public launch of ChatGPT, has only increased the threat vector. Understanding this phenomenon helps in devising strategies to counter such attacks and safeguard your AI models from hostile data manipulation.
Data Utilization in ML Model Training
Training an ML model requires access to large amounts of data, referred to as training data, from a variety of sources. Some prevalent sources include the internet (containing blogs, social media platforms, news sites, and more), IoT devices‘ log data, scientific publications, government databases, specialized ML repositories, and company proprietary data. Data poisoning attacks occur when threat actors infiltrate the training data, causing the AI model to operate inaccurately or sabotage its overall performance.
Types of Data Poisoning Attacks
Data poisoning is executed in a variety of ways. A common few are Mislabeling attack, where wrongfully labeled data creates false patterns in the model; Data injection, where threat actors smuggle damaging data samples into models for biased outcomes; Data manipulation, where modifying the training set data results in misclassification and biased results. This can be accomplished by adding wrong data, removing right data, or integrating adversarial samples.
Hidden Threats: Backdoors and Supply Chain Attacks
Moreover, there’s a technique of planting concealed vulnerabilities known as backdoors, either in the training data or the ML algorithm itself. These are triggered under specific pre-set conditions, forcing the model to yield malicious results. Backdoor attacks can be undetectable as the model seems to behave normally after deployment. The threats are not just confined to these. ML systems can also be vulnerable to playing a part in Supply chain attacks, which can infiltrate at any stage of the ML system development cycle.
Insider Attacks: Threat from Within
Another threat is the insider attacks, which are essentially acts of sabotage by people within the organization who misuse their privilege to access ML model’s data, algorithms, and infrastructure. Since these insiders can bypass external security controls, these attacks are especially dangerous and difficult to defend against.
Direct vs Indirect Data Poisoning Attacks
Data poisoning attacks can be also classified into two types based on their objectives: direct and indirect. Direct attacks aim at manipulating the ML model’s reaction to a particular targeted input without affecting its general performance. An indirect attack, conversely, aspires to impact the ML model’s performance as a whole.
Strategies to Mitigate Data Poisoning Attacks
To effectively combat data poisoning attacks, organizations must implement a multi-layered defense strategy that integrates best security practices and access control mechanisms. A few mitigation techniques include validating training data, constant performance monitoring and auditing, access control to the ML model, adversarial sample training, and fostering diversity in data sources.
In addition, maintaining a record of training data sources and access tracking (users and systems that access the model and their activities) is a crucial step in identifying potential threat actors and thus helpful in mitigating many types of poisoning attacks.
Closing Remarks
With our bespoke AI and ML models, HAL149 can help businesses improve operational efficiency, amplify growth potential, and counteract potential data poisoning attacks. Don’t hesitate to contact us today.