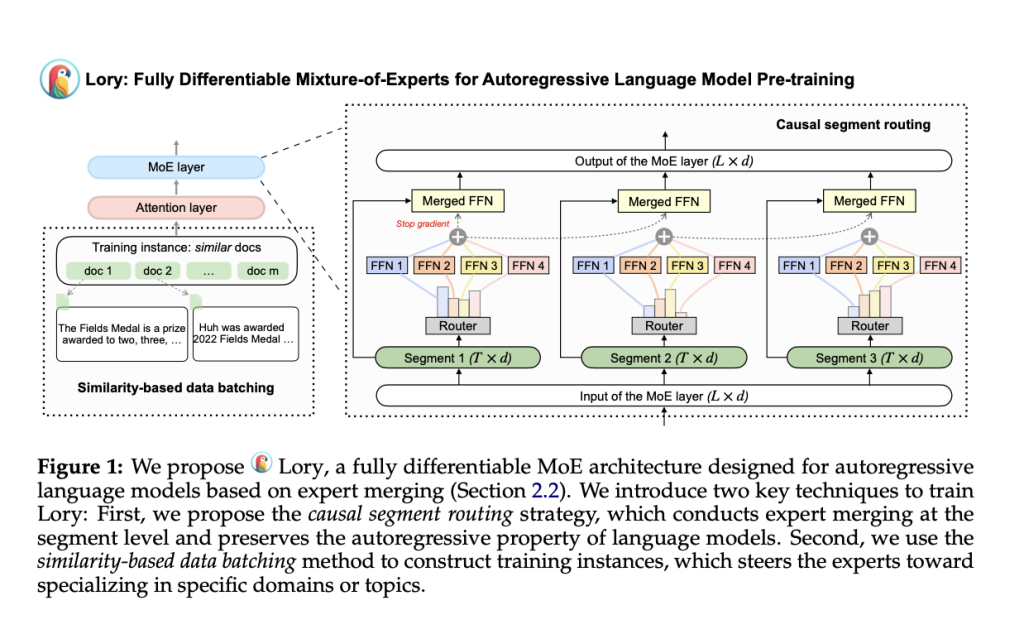
Mixture-of-experts (MoE) Architecture: An Overview
In the realm of modern artificial technology, Mixture-of-Experts (MoE) architectures have leveraged sparse activation for the efficient scaling of model sizes while maintaining high-quality training and inference outcomes. Despite the substantial gains achieved by MoE models, training the router network poses significant challenges, including optimizing a non-differentiable, discrete objective.
Recently, the emergence of the SMEAR architecture, a fully non-differentiable MoE model, has sought to simplify this process by softly merging experts in parameter space. While effective in small-scale fine-tuning experiments, the application of SMEAR has largely been restricted to downstream classification tasks.
Scaling MoE Models: Lory
Recognizing the potential of MoE models, researchers at Princeton University and Meta AI introduced a method to augment these architectures in an autoregressive language model pre-training context — Lory. Consisting of two primary techniques, Lory aims to facilitate optimal routing operations while supporting the natural autoregressive nature of language models. Lory’s uniqueness stems from its similarity-based data batching method which significantly aids expert specialization by grouping similar documents throughout the training process. The use of token-level routing, as opposed to segment-level routing, further improves the overall performance of the model.
The principle techniques underpinning Lory include casual segment routing and a data batching method based on textual similarities. The first, casual segment routing, works by decomposing longer sequences of input tokens into smaller segments at a fixed length. Following this, the router’s weight originates from the original segment, subsequently leading to the merged expert for the next segment.
With an increase in the merging of random document sets in text data for language model pre-training, expert specialization risks being compromised. Consequently, the second technique, similarity-based data batching, identifies and groups similar documents to create sequential segments during the training process, leading to more efficient training for expert routing.
Why Choose Lory?
Lory’s benefits become particularly evident in key areas such as:
- Efficiency in Training and Convergence: Lory achieves an equivalent loss level at half the training tokens for 0.3B and 1.5B models, indicating more superior performance despite the same training compute.
- Language Modeling: Lory’s proposed MoE models outperform their dense counterparts across all domains, leading to a decrease in perplexity.
- Downstream Tasks: The 0.3B/32E MoE model delivers improved average performance results in common sense reasoning, reading comprehension and text classification tasks.
Future Prospects of Lory
The future focus of this breakthrough is centered on scaling Lory and integrating token and segment-level routing through developing efficient decoding methods for Lory. As such, the researchers envision that Lory will continue to represent a significant stride in advancing Mixture-of-Experts (MoE) architectures.
For further explorations of Lory, please refer to the Original Research Paper.
How Can HAL149 Help?
HAL149 is a pioneering AI company offering bespoke, AI-assisted solutions to businesses. By automating substantial online tasks, HAL149 enables companies to utilize their growth potential more efficiently. To learn more about how HAL149 can empower your enterprise, visit https://hal149.com or get in touch via email at hola@hal149.com.