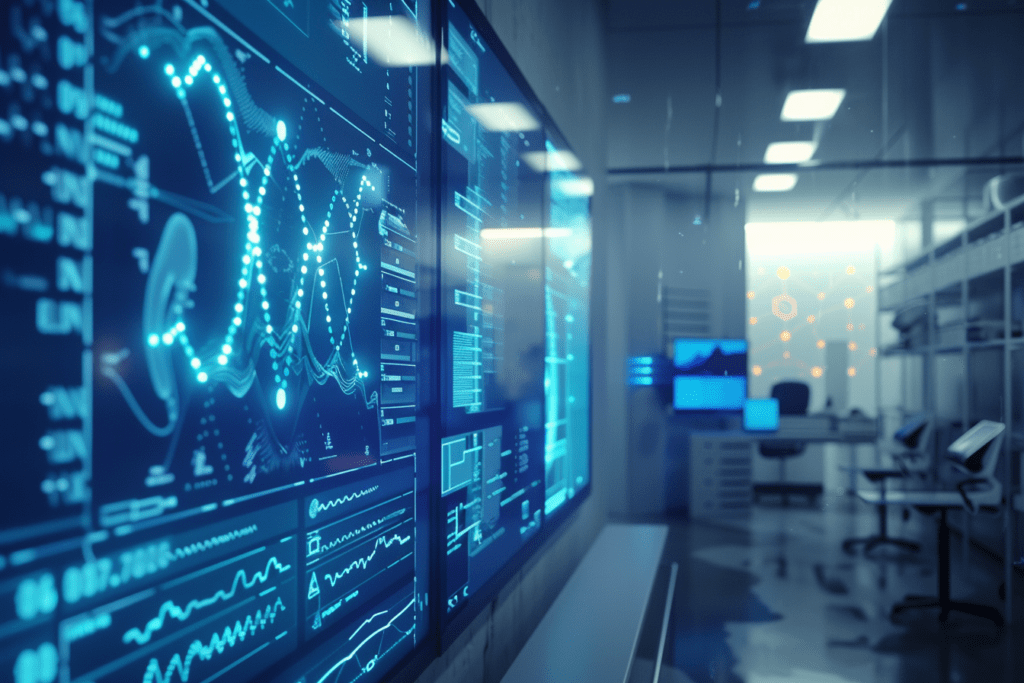
The Power of AI in Harnessing Data Registries
The medical landscape has been swept up in the rapid rise of technological advancements, including the utilization of natural language processing (NLP), artificial intelligence (AI), and machine learning (ML). From interpreting the onslaught of medical data to assisting in patient care, the potential benefits of AI applications are vast. Nonetheless, their introduction also presents certain risks and challenges.
Data Interpretation with NLP, AI, and ML
Healthcare institutions churn out immense volumes of data daily. Harnessing the power of data through AI and machine learning algorithms has become an integral part of the healthcare industry’s efforts to streamline its operations, improve patient care and efficiency, and manage clinicians’ workload. Through the applying tools like NLP, AI, and ML, medical institutions can tap into the potential use cases for AI, such as boosting patient care, refining clinical trial operations, and automating clinical workflows.
Regulating AI Usage
Although the implementation of AI holds potential to reform healthcare, there is an essential need for comprehensive frameworks and guidelines. Organizations, including the World Health Organization and government bodies, emphasize the need for responsible AI application in healthcare. Businesses in heavily regulated industries like healthcare must tread cautiously with their use of AI.
AI in Clinical Registries
Integrating AI in clinical data registries can significantly tackle the issue of high variability in data formats and extraction of useful insights from large, diverse datasets. AI can be employed for identifying, extracting, encoding, and analyzing data, enhancing the value of the registry. However, the utility of AI in clinical registries depends on appropriate application – improving care quality, aiding research, and de-identifying datasets, but must be abstained from calculating outputs submitted to regulatory authorities.
Employing AI to Improve Care Quality
Unstructured data, such as free-text notes, form a significant portion of clinical data registries. AI is incredibly beneficial for extracting, analyzing, and drawing insights from this type of data, which can pave the way for targeted quality improvement initiatives. For example, data could be used to identify how many opioid poisoning or overdose patients were prescribed naloxone at discharge. AI can also derive insights from structured data elements that can help to drive consistency in clinical decision-making across practices to improve patient health outcomes.
AI in Support of Research
Medical research initiatives stand to gain from the wealth of healthcare data available through clinical data registries. Machine learning models can use this data to support the risk stratification of patient populations. By identifying disease trajectories and predicting adverse events, this data can inform clinical decision-making and innovative treatment approaches.
De-Identification of Datasets Using AI
AI algorithms can significantly accelerate the process of data de-identification, which is often a requirement for certain applications such as monitoring adverse events, designing clinical trials, and informing drug prices. One study demonstrated that NLP systems outperformed human efforts in identifying and removing protected health information (PHI) from clinical notes. However, the use of AI for de-identification carries risks, and it’s crucial to manage them properly to prevent violations of patient trust and financial penalties.
Limitations and Risks of AI
Qualified Clinical Data Registries (QCDRs) bring value by simplifying the process of quality reporting. Nevertheless, using AI to extract and encode data for the purpose of calculating these measures may lead to false positives or false negatives, due to their probabilistic nature, which can result in incorrect scores. This introduces significant audit risk, and hence caution must be exercised when submitting data to regulatory authorities.
Additionally, AI technologies may also produce inaccurate outputs through hallucinations, especially when decisions about healthcare are involved. Lastly, transparency plays a critical role in maintaining patient trust when using AI in healthcare.
In conclusion, AI tools present valuable opportunities for healthcare companies in analyzing and interpreting health data, and enabling a range of use cases. However, considerations about patient trust and regulatory audits are of paramount importance. It is essential for the healthcare sector to ensure responsible and appropriate use of AI.