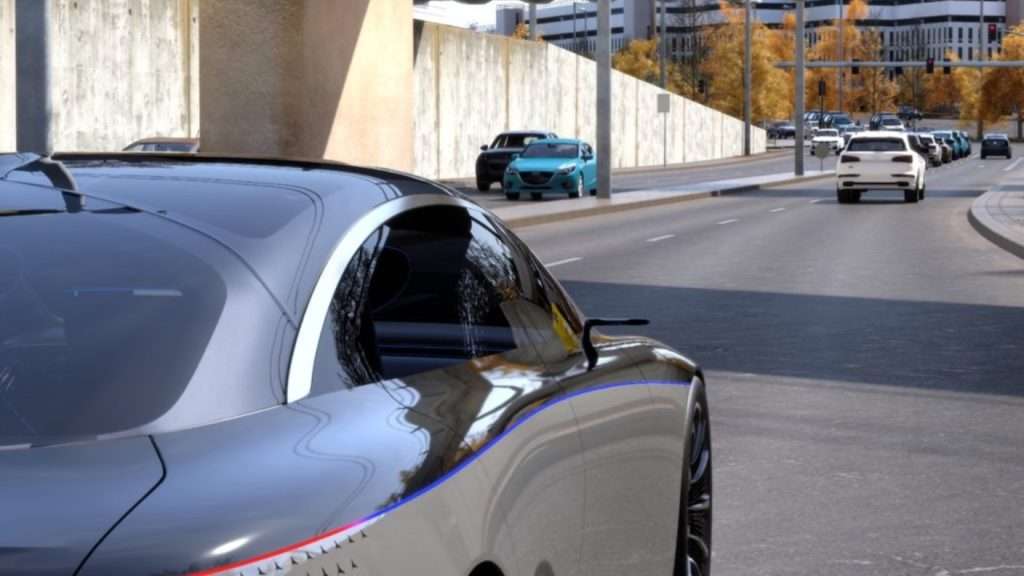
The Role of Simulation in Training Autonomous Vehicles
It’s no secret that autonomous cars need practice. Every year, these robotic taxis spend millions of miles on public roads, using sensors such as cameras, radar, and lidar to gather essential data. This data helps train the neural networks responsible for their operation.
For those residing in cities where autonomous cars run, the frequent sight of these self-driving cars gives an idea of the amount of practice needed.
The Rise of Computer Simulation in Training
There has been a remarkable evolution in computer graphics, resulting in a higher degree of realism and fidelity. This transformation is why industries are increasingly turning to simulation to expedite the development of their algorithms.
For instance, Waymo says its autonomous vehicles have already driven about 20 billion miles in simulations. Be it industrial robots or drones, these machines rely significantly on these virtual worlds to gather the required data and practice hours.
The Advantage of Simulation
According to Gautham Sholingar, a senior manager at Nvidia who specializes in autonomous vehicle simulation, simulations offer a unique benefit—they allow for the consideration of uncommon scenarios it’d be nearly impossible to account for in the real world.
Real-life scenarios that involve risks to pedestrians and other road users or situations that challenge correct measurement such as determining the velocity of far-off objects pose immense challenges for data collection. This is where simulation outperforms, as Sholingar explained in an interview for Singularity Hub.
Simulation and The Artificial Intelligence Lens
Improvements in computing power, the ability to model complex physics, and the evolution of GPUs (Graphical Processing Units) that power today’s graphics underscore the growing utilization of simulated worlds for AI training.
The quality of graphics is fundamental due to how AI “interprets” the world. As graphics rendering technology edges closer to photorealistic standards, distinguishing between images captured by real-world cameras and those created in a game engine becomes almost impossible.
Enter Nvidia’s Omniverse
Nvidia, leveraging their proficiency in GPUs, has positioned itself at the forefront of this space. In 2021, the company debuted Omniverse, a simulation platform capable of rendering top-quality synthetic sensor data and simulating real-world physics essential to a range of industries.
Developers are now utilizing Omniverse to generate sensor data for training autonomous vehicles and robotic systems.
The Future of Simulation in AI Training
Clearly, the steady improvement of physical and graphical engine technologies means that virtual arenas provide a valuable, low-risk sandbox for machine learning algorithms. These virtual worlds enable the creation of functional tools that will drive our autonomous future.
While real-world training and testing remain indispensable to the development of autonomous systems, synthetic data generated through simulations will continue to augment datasets collected from real-world scenarios.
Shaping the World of Autonomy
The bigger landscape of aiding “things that move” in achieving autonomy is gradually shifting towards simulation. Tesla, Microsoft, and the Canadian startup, Waabi, are already integrating similar technologies into their developmental processes.
Sholingar emphasizes that what matters in simulation isn’t just the visual appeal but the accuracy in representing content, behavior, and appearance. When these three aspects are accurately depicted, simulation becomes realistic. Simulation doesn’t replace real-world data collection—it accelerates it. In other words, it’s a tool that supplements real-world data collection.
Image Credit: Nvidia